Beyond Gen AI: Navigating the Multifaceted Landscape of AI in Marketing Insights
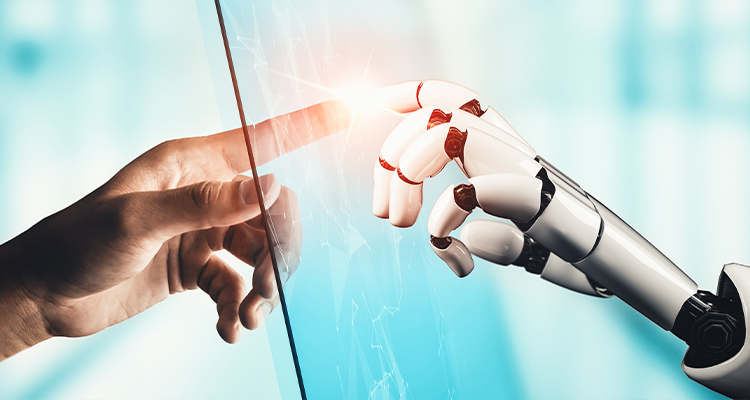
In our experience as practitioners of marketing research, we have noticed a common misconception surrounding Artificial Intelligence (AI) platforms and tools: many equate AI withGenerative AI (Gen AI). While it is true that many platforms do use Gen AI, the reality is that in the realm of AI applications, particularly in marketing research, the diversity and application of AI technologies go well beyond Gen AI.
At Q2 Insights, our approach involves leveraging a variety of AI technologies in conjunction with human researchers. Our AI platforms serve as unique tools for data gathering and comprehensive initial analysis. This initial AI data analysis is then augmented by expert human analysis, creating a powerful combination. These AI-driven platforms, which go beyond Gen AI, encompass:
Clustering
This involves grouping data points into clusters to inform targeted marketing strategies. In marketing research applications AI Clustering might include:
Thematic Clustering: Grouping text data such as qualitative research from focus groups or survey open-ends, into coherent themes or topics.
Auto-Coding: Automatically categorizing qualitative data, like open-end survey responses, into predefined or emergent categories a process traditionally performed manually known as open-end coding or tagging.
These types of AI Clustering are invaluable across a spectrum of marketing research applications such as: Customer Segmentation, Market Basket Analysis, Behavioral Analysis, Churn Prediction, Campaign Effectiveness, Geo demographic Clustering, Product Development, and Competitor Analysis.
Sentiment Analysis
Primarily, this entails classifying text as positive, negative, or neutral. Sometimes, Sentiment Clustering is used to unearth nuanced patterns and themes within sentiment categories, providing deeper insights into customer opinions and emotions.
Sentiment Analysis finds numerous applications in marketing research, spanning Brand Monitoring, Customer Feedback Analysis, Product Development, Competitive Analysis, and Advertising and Campaign Analysis.
Collaborative Filtering
This involves personalizing recommendations based on user behavior. AI automatically predicts user interests by aggregating preferences or tastes from a multitude of users.
Encoders for Natural Language Processing (NPL)
These encode text data into numerical form, enabling machine learning models to process it. Encoding the data can provide valuable insights that can be extracted from large volumes of text data unveiling sentiments and emerging trends to guide data-driven decisions.
TF-IDF (Term Frequency-Inverse Document Frequency)
This statistical measure, utilized in NLP, extracts pivotal terms from documents for text analysis. Examples include Keyword Analysis and Content Classification.
Translation AI
An example of this is Google Cloud Translation API which leverages Machine Learning and Natural Language Processing (NLP) to translate text across languages, grasping contextual nuances in the process.
Conversational AI
Facilitating natural language interactions between humans and computers, Conversational AI, often embodied in chatbots, represents a swiftly evolving domain with enormous potential. In marketing research, applications extend to survey automation, feedback analysis, and qualitative interviews.
Emotional AI
Emotional AI is all about understanding and responding to human emotions through sophisticated data analysis. It covers a wide spectrum, from recognizing emotions based on facial expressions to analyzing voice tones and even dissecting textual cues. This field is teeming with potential, especially in the realm of marketing research, promising insights that transcend mere data points.
The vision? Creating chatbots and virtual assistants capable of engaging in emotionally intelligent conversations, mirroring human interaction. Picture this: interviews and surveys conducted in a fraction of the time it takes for humans yet maintaining that crucial human touch. While we have seen remarkable progress in this area, it is important to note that the technology is still evolving. There is still ground to cover before EmotionalAI is perfected.
How is Generative AI Different from Other Types of AI?
Generative AI, leveraging partnerships with OpenAI and LargeLanguage Models (LLMs), excels in crafting new content across various modalities, from text to images. Unlike other types of AI outlined in this article, Generative AI's primary focus lies in generating novel content rather than analyzing existing data or making predictive assessments based on patterns. Its emphasis on content generation, versatility, and potential for creativity and innovation sets it apart in the AI landscape. This unique capability enables Generative AI to offer deeper research insights, facilitate data augmentation, and support scenario planning across diverse domains.
The Whole is Greater Than the Sum of its Parts
While each form of AI described in this article holds significant utility in marketing research and other disciplines, it is the unique combination of some of these tools within each of Q2 Insights' platforms that amplifies the platform’s power and earns appreciation from our clients.
Conclusion
In navigating the dynamic landscape of AI applications in marketing research, it is crucial to recognize that while Gen AI serves as a pivotal and popular tool across disciplines, construing it as the sole form of AI in play would be a misstep.
Kirsty Nunez is the President and Chief ResearchStrategist at Q2 Insights a research and innovation consulting firm with international reach and offices in San Diego. Q2 Insights specializes in many areas of research and predictive analytics, and actively uses AI products to enhance the speed and quality of insights delivery while still leveraging human researcher expertise and experience.