AI Versus Manual Open-End Coding: Efficiency, Accuracy, and Insights

During a recent quantitative study at Q2 Insights, we had the opportunity to compare three different approaches to open-end coding using the same dataset:
- Manual open-end coding
- AI-driven open-end coding through a survey platform that uses Large Language Models (LLM) to generate insights in both text and graphics
- AI-driven coding via a standalone tool that leverages LLM and GenAI to parse open-ended comments, cluster into topics and present summary findings, insights, and visuals
As expected, the AI-driven methods outperformed manual coding in terms of speed, cost, and accuracy. The dedicated AI tool was particularly effective for its specific task, but this does not take away from the broader capabilities and versatility of the AI-powered survey platform we used.
What is Open-End Coding?
In the past, coding responses to open-ended questions in surveys or qualitative research like interviews or focus groups was a time-consuming, expensive process. Open-ended questions allow for unstructured responses, which adds complexity. Analysts had to create codes for every response category, often leading to multiple columns of codes per question. This process required a “code frame” that assigned numbers to each response and had to be refined continuously before analysis could begin. When done manually, open-end coding was, and still is, a bit of a nightmare.
Here is an example of what the open-end coding might look like:

Enormous Variability in the Quality of Manual Open-End Coding
A major challenge with manual coding is the inconsistency in quality. The output depends on the skill and attention to detail of the analysts performing it. When done well, manual coding can provide rich insights. However, if done hastily or without care, it leads to shallow and unreliable analysis. Even with experienced analysts, human error, fatigue, sloppy coding, and subjective interpretation introduce variability, making manual open-end coding challenging, particularly in large-scale studies.
AI Open-End Coding Addresses Human Variability
AI-driven coding resolves many issues with manual coding by ensuring speed, consistency, and reduced human error. AI can analyze large volumes of open-ended responses, applying the same rigor to each, and quickly identifying themes and patterns that would take human analysts far longer. This allows researchers to focus more on interpreting insights rather than the labor-intensive task of coding. Human researcher review and oversight is still recommended; however, this will generally be minimal.
AI tools can also flag unusual or complex responses for human review, ensuring that important nuances are not missed. While AI may not fully replace the insight and interpretation skills of human analysts, it dramatically streamlines the process and makes coding more scalable and efficient.
Example of Differences Between AI Approaches
In one study, we focused on The Navy SEAL Museum San Diego, set to open in 2025, and an existing museum in Fort Pierce, Florida, the Navy SEAL Museum, and compared AI-driven approaches to the open-end question, “What do you imagine The Navy SEAL Museum would offer visitors?”
- The AI survey platform generated detailed, colorful graphics, allowing users to drill down on high-level findings, with themes such as “Exhibits” and “Interactive Experiences.”
- The standalone AI tool summarized themes in paragraphs without interactive visuals, identifying high-level themes like “History,” “Artifacts,” and “Comprehensive Collection.” Its unique deliverable included rich narrative descriptions of these themes, providing deeper context to each finding.
The AI platform open-end coding delivered the following findings. The graphic is provided for illustration purposes only. The actual graphic delivered by the platform was not only more visually appealing, but it was also interactive, allowing users to drill down on the higher-level findings. In the graphic below, the orange-colored bars represent Themes, and the teal bars represent Representative Statements. The % next to option is Expected Support, which is an AI based calculation based on the estimated % of the sample that would agree with that statement/theme. The % next to bars is % of total responses.
What The Navy SEAL Museum Would Offer Visitors
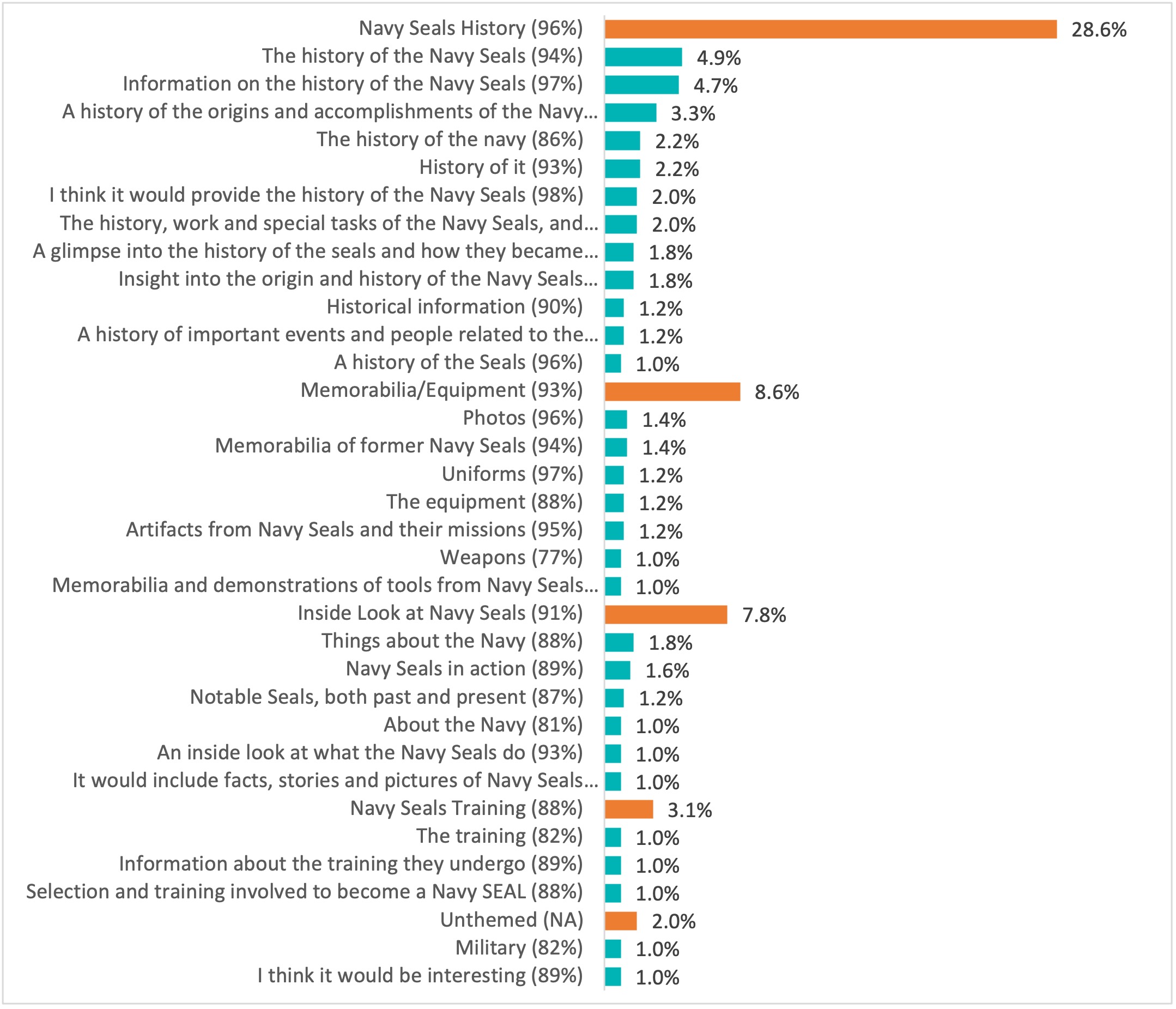
The AI open-end coding through a standalone tool provided a graphic of the top-level categories of comments and a detailed description of each topic in paragraph form. Interestingly, the high-level themes were different from those of the AI-driven open-end coding through a survey platform. The image below shows the sub-topics for one topic, “interesting place to visit,” along with summaries for each, including sentiment counts.

One of the deliverables from the standalone AI tool that is different from the AI-driven open-end coding through a survey platform is a summary of the responses included under each topic in paragraph form. Here are some examples:
History
The history of the Navy SEALs is likely to encompass the organization’s inception, evolution, and significant accomplishments. It will delve into the formation of the elite unit, its heroic missions, and the sacrifices made by its members throughout history. Also included would be the Navy SEAL’s rich heritage, their role in shaping naval history, and their contributions to national security.
Historical Artifacts
The Navy SEALs history and artifacts would include a wide range of historical items, relics, and information related to the Navy SEALs, an elite special operations force of the United States Navy. Visitors will be able to explore exhibits highlighting the group’s history, achievements, and significance in the U.S. military over the past century. The museum will house historical artifacts, photographs, and displays that provide insight into the origins and evolution of the Navy SEALs, including their involvement in significant events such as World War II. Additionally, the museum will likely offer information on famous Navy personnel, uniforms, weapons, and equipment used throughout history, as well as memorials honoring great naval officers.
Comprehensive and Immerse Experience
Consumers believe the Navy SEAL Museum will offer visitors a comprehensive and immersive experience, displaying the rich history, accomplishments, and sacrifices of these elite forces. Through interactive exhibits, displays of equipment, and tributes to fallen heroes, the museum will educate and inform visitors about the Navy SEALs’ rigorous training, heroic missions, and legacy. It provides a glimpse into the history and origins of the Navy, allowing visitors to explore the various areas in which these teams operate. The museum will also likely offer a chance to see the Navy SEALs’ memorial and metals, as well as learn about the history of the United States Navy SEALs through interactive exhibits.
Summary
This article compares manual open-end coding with two AI-driven approaches (through a survey platform and a standalone tool). The study revealed that AI-driven methods are faster, more consistent, and less expensive, with the standalone AI tool excelling in specialized tasks while the survey platform demonstrated broader capabilities. Manual coding, while potentially insightful, carries significant variability in quality due to human factors, a challenge AI helps mitigate.
Kirsty Nunez is the President and Chief Research Strategist at Q2 Insights a research and innovation consulting firm with international reach and offices in San Diego. Q2 Insights specializes in many areas of research and predictive analytics, and actively uses AI products to enhance the speed and quality of insights delivery while still leveraging human researcher expertise and experience.